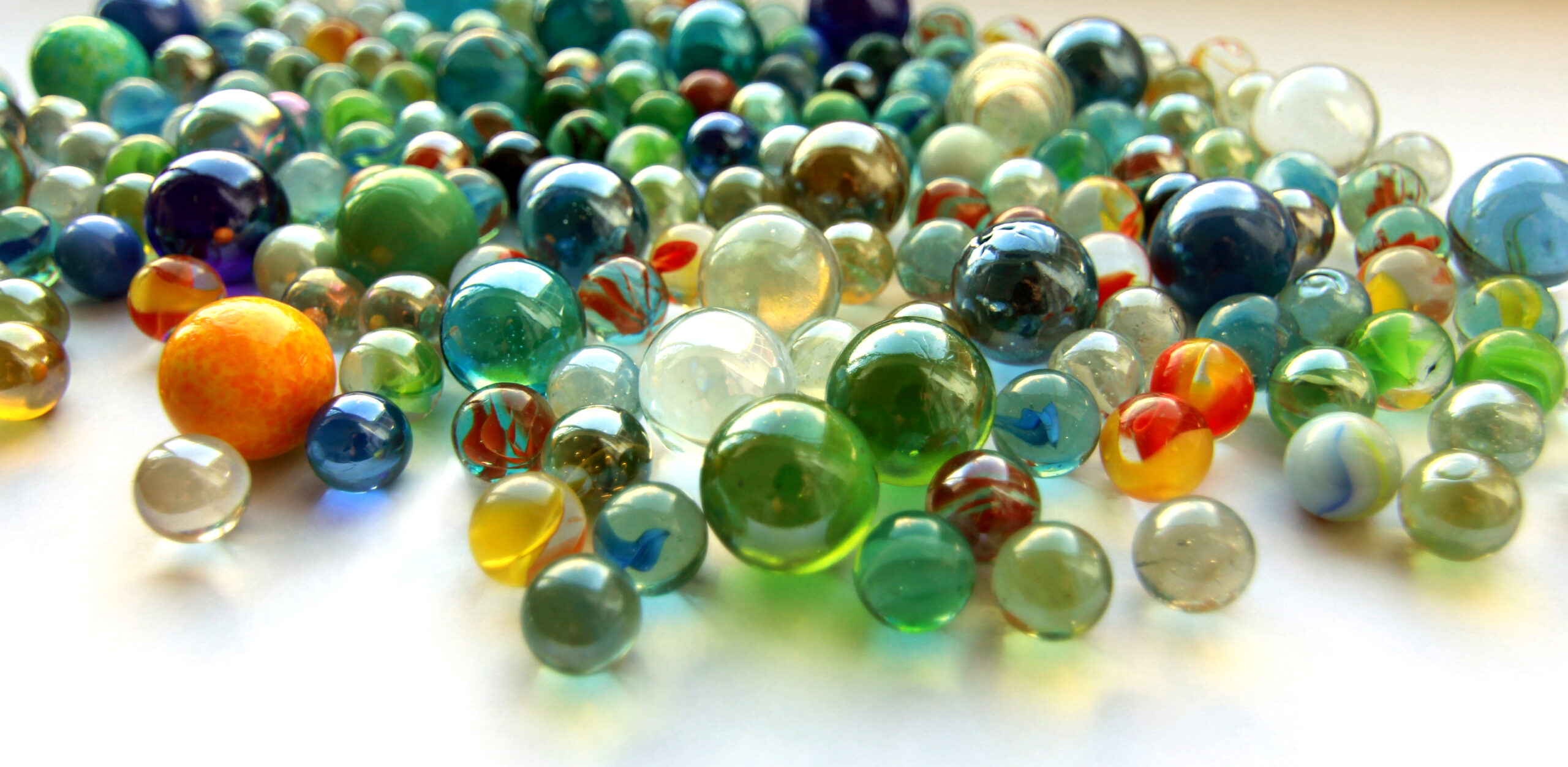
Building a better retention model: Uncommon variables add up to multi-million dollar savings
Published 6th Apr 2015It’s cheaper to keep a customer than find a new one, goes the old business adage. And it’s true, from both a cost and a time perspective.
Before the advent of retention models, insurers had to figure out which of their millions of policyholders were mostly likely to defect. Agents would call all of their renewing policyholders each month assuming every last one had doubts about renewing. Today they might call only a small portion– those singled out by a retention model that predicts those least likely to renew and those on the cusp, who could be persuaded to renew through a loyalty program or other incentive.
As more insurance companies awake to the potential of big data analytics, they can become more targeted and efficient in their customer retention as long as they use accurate data, which offers better insights and can be more precise as predictive variables. For instance, better understanding the financial health of a household or business might enable insurers to better pinpoint policyholders most likely to move their insurance policies to a competitor.
But what variables best predict a customer on the fence or tempted by the overtures of a worthy competitor? We partnered with a top 25 insurance carrier to find out, testing a set of variables selected from the more than 200 in the Powerlytics database to determine their ability to predict retention. By comparing the insurer’s current model with a Powerlytics retention model, we were able to see how different variables could help insurers hone their list of most vulnerable customers. Here’s what we found.
Uncommon variables, new insights
The insurance carrier’s current retention model was built on 18 variables, including rate change, and spanning everything from policy and property to billing, agent, and territorial characteristics. The carrier tested an additional 148 variables, and found that 25 of them were predictive of customer loyalty.
Most of the retention models used in the insurance industry include many common variables. For example, the more lines of insurance a customer has with a particular carrier, the more loyal that customer is likely to be. In this study we tested uncommon variables, metrics not likely to be a part of most retention models. Among the 25 that were found to have predictive power were non-wage income as a percentage of disposable income, percentage of tax filers who use paid preparers, and even the percentage of tax filers who gave gifts to charity. Powerlytics proprietary Business Risk score was also shown to be predictive of customer retention.
Compared to the insurer’s existing retention model, when Powerlytics’ variables are added to the model they provided greater lift as well as fewer errors between the prediction and the actual result. By implementing proactive and assertive retention strategies, the insurer would experience a 0.19 percent incremental policy growth above the current model using the Powerlytics model.
The impact of a better retention model
That small percentage can have a significant impact. An insurance carrier with approximately 2 million homeowners policies could retain an additional 3,800 policies using the Powerlytics model. At an average premium of $1,000, that equates to an incremental benefit of $3.8 million dollars.
In addition, the insurance carrier we worked with already had a finely tuned and effective retention algorithm. A carrier with a less effective retention model could see even greater lift and savings.
By integrating uncommon variables around financial health into their retention models, insurers can not only retain more customers, they can also grow without writing as much new business. Better data enables clearer insights into policyholders, which in turn can help insurers improve service and strengthen relationships across their organization.
Read next
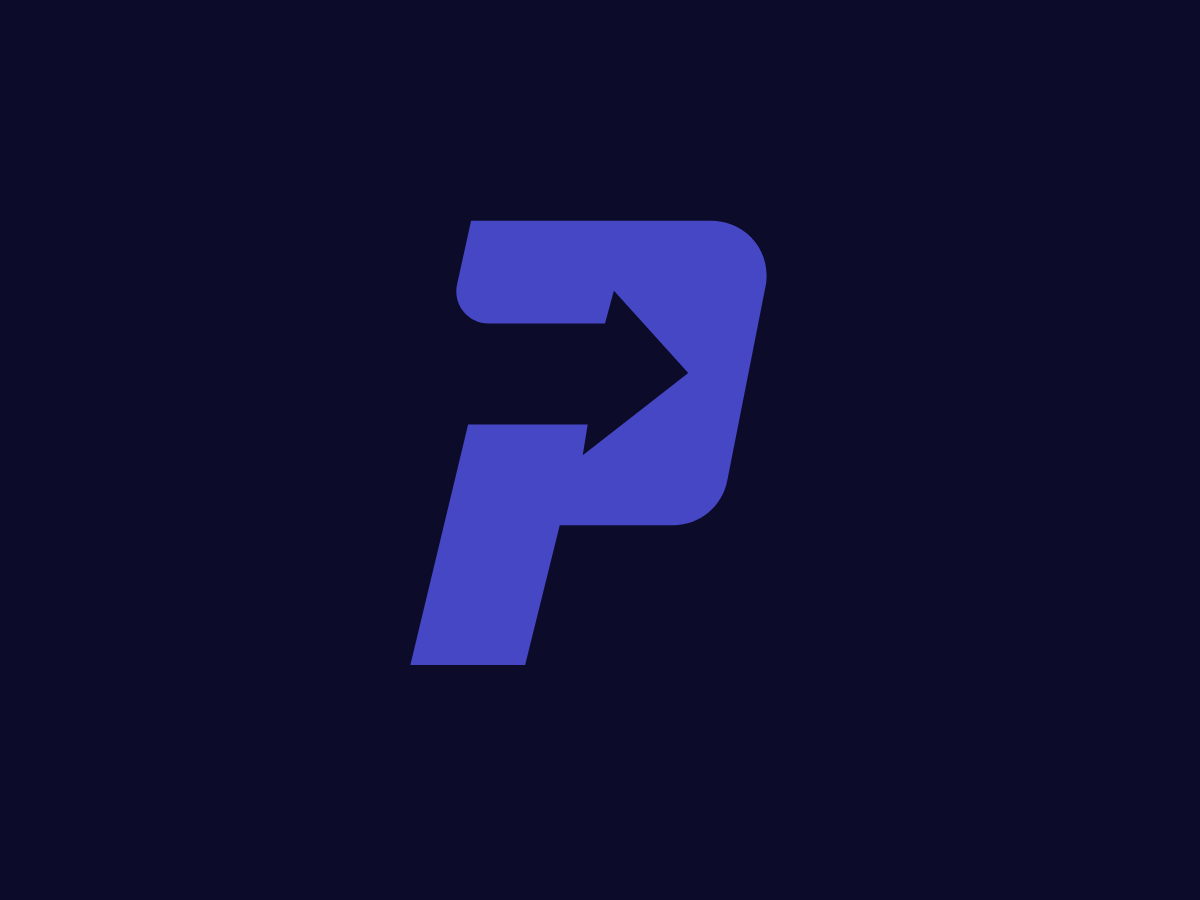
Published 13th Mar 2015
ArchivedHow Property and Casualty Insurance Professionals Can Find the Truth in Big Data
If you’re not yet using big data and analytics to do your job, you likely will be soon. Surely you’ve heard of big data transforming industries as varied as medicine, advertising, and...
Read Article