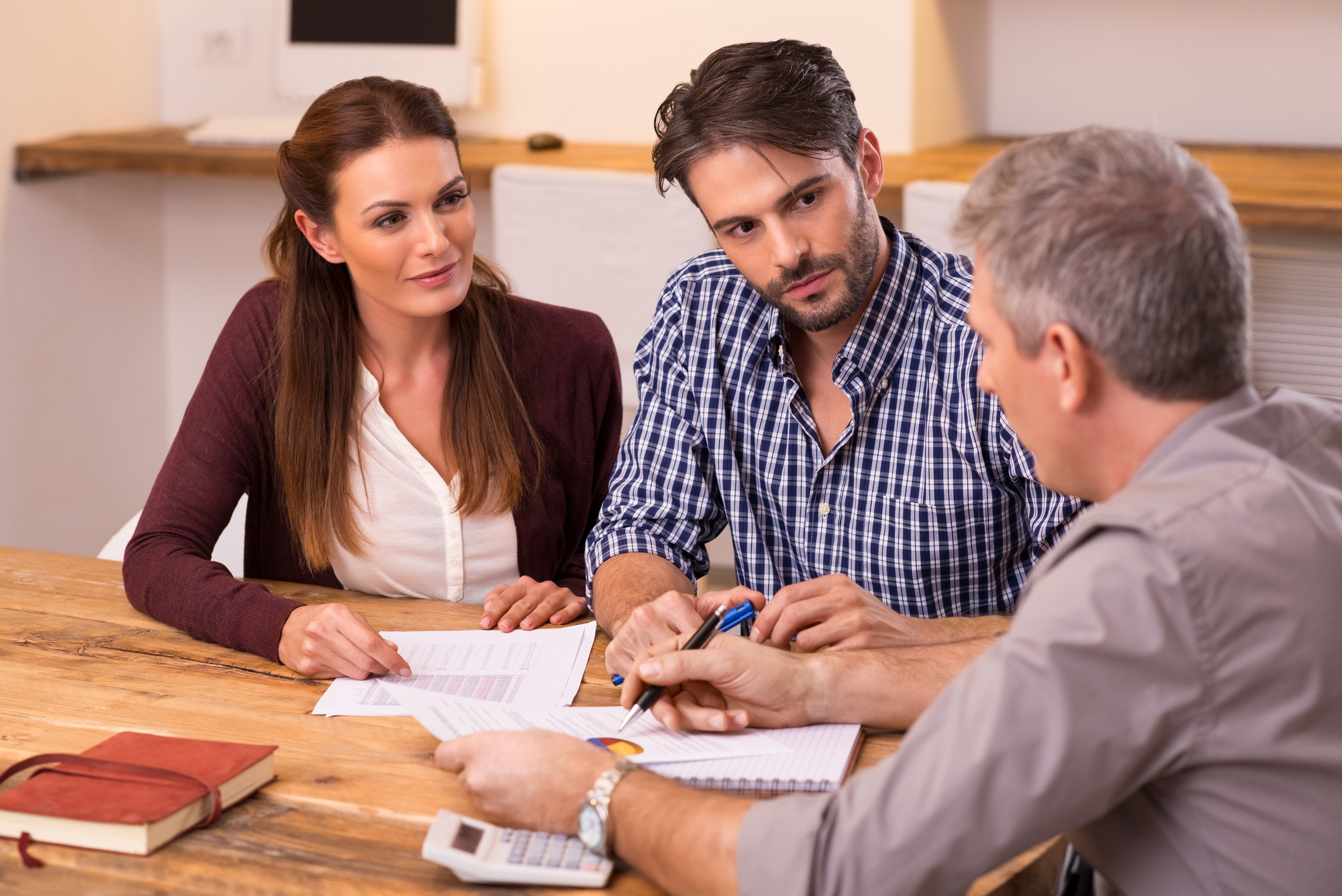
The secret to improving insurance retention
Published 19th Jan 2017For insurance companies, finding new customers is expensive. By some estimates, advertising and commissions can cost between $500 and $800 per policy.
That’s why insurance companies are laser-focused on retaining current customers, and dedicating significant resources to identifying which customers in their book of business are most likely to leave. Even small upticks in the percentage of customers who stick around can yield big results.
When we provided a top five auto insurance carrier with data for their retention model, it was estimated that improving retention rates by only 0.5 percent could save 60,000 policies and $108 million in premium.
Typically, when carriers develop a retention model, they will rely on data that is collected during the application process and found on their customers’ policies. This data includes information specific to the individual or vehicle, such as age, marital status, driving record, vehicle make/model and policy limits. An insurer may find, for example, that customers who carry the minimum amount of insurance coverage are more likely to leave.
In the competitive insurance market, insurers are continually looking to improve their predictive models by finding new data variables that better identify whether a customer may leave. To achieve that, many are turning to external data vendors with aggregated data to supply insight about their customers.
For example, a top five insurance carrier developed two new retention models using Powerlytics’ financial data variables at the Zip+4 level. Their intention was to see whether either model would perform better when compared to the one they had been using.
The first model, using only Powerlytics’ data variables, increased the reliability of its predictions by 5 percent. It’s amazing that the customer built a better model than the original without using any customer-specific information. A combined model, built from both Powerlytics and customer-specific policy data, beat its existing model by 6.7 percent.
While clearly, a customer’s financial state is one of the strongest indicators of whether they are likely to renew their policy, an insurer can rely on using the Powerlytics financial data at the Zip+4 to achieve improved model results.
One data variable that proved highly predictive of whether someone was likely to leave was the percentage change of people filing single on their taxes. This intuitively makes sense. When someone gets married or divorced, they tend to shop for insurance. Another data variable that was predictive of a customer leaving was the ratio of business income to adjusted gross income. If a greater percentage of your income comes from an unpredictable monthly income stream like a business, as opposed to a stable income stream like W2 Wages, you may be more price sensitive and therefore more likely to leave.
Insurance companies use retention models to increase the efficiency of their retention strategies. With a retention model, the carrier can rank their customers according to their likelihood to leave and devote extra resources to retaining customers in the highest tier. But because the model leveraging our data does not rely on any customer data, it can be used to predict retention at the time the carrier offers a quote to a prospective customer, and it can even factor into its overall new business pricing strategy.
For almost any company, there’s a lot that can be done with internal customer data. That approach, however, has its shortcomings. Customers make decisions for reasons that cannot be collected on an application. Powerlytics data has the ability to supplement internal data, resulting in a more complete view of customers which can quickly turn into increased revenue and profits.
Read next
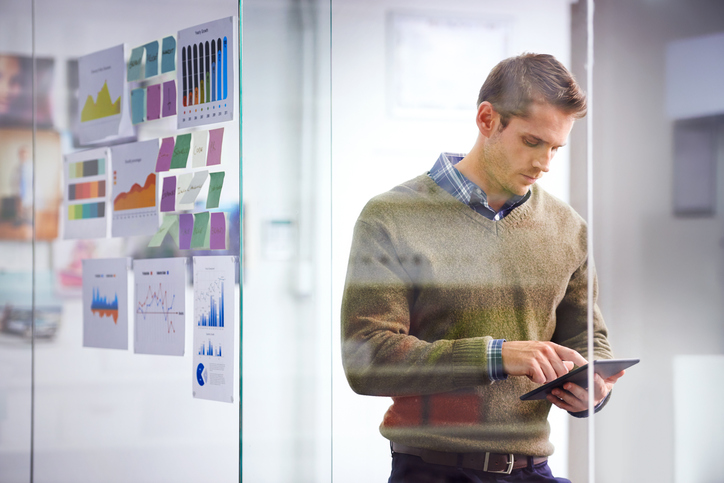
Published 9th Dec 2016
ArchivedWhat most businesses get wrong about market segmentation
Market segmentation is one of the truly powerful tools to come out of the big data revolution, but in too many instances, its promises fall far short of its capabilities. Many businesses...
Read Article