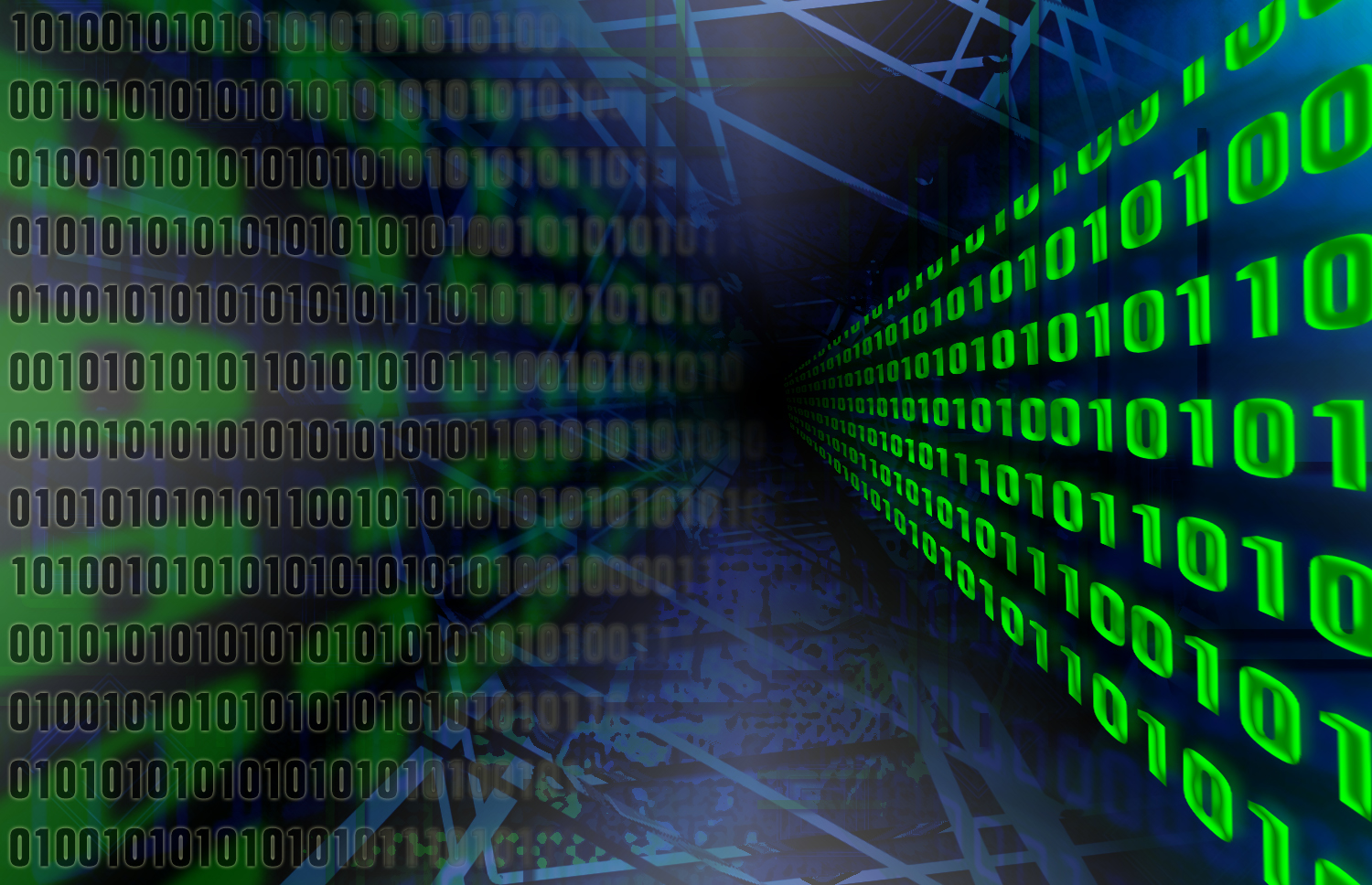
Big Data Meets “All” Data
Published 22nd Dec 2014When we published the results of our proprietary study of the most profitable industries in the United States, to our knowledge, it was the first time anyone conducting this type of research had ever analyzed the entire subject population. Frequently, studies of this nature are based on surveys or samples, both of which are notorious for their errors. So, when we published a study that included every single for profit business in the U.S., it represented the realization of a vision that traces its roots back more than ten years.
The inherent flaws of samples and surveys and the obvious need for simply better data provided the motivation to create Powerlytics. Both Jose and I had spent a good portion of our careers in positions where we witnessed firsthand the use of flawed data and how it was affecting decisions. From the availability of credit to the products on the shelves of our local market, businesses were increasingly relying on data to make these important decisions, and most of the data was incomplete at best and in many cases just plain wrong.
Jose first became interested in big data when he was conducting academic research that eventually grew into consulting with key government agencies. That’s where he began to understand how most data was incomplete, inaccurate and of poor quality – causing frustration and an inability to make the best business decisions. Meanwhile, I was seeing how financial institutions where using data to underpin decisions and help create knowledge-based products and global processes for KPMG – one of the largest audit and consulting firms in the world. Much of this was looking at how to better measure, monitor and control risk as well as understand the market dynamics that drive success for businesses.
Powerlytics was born when we realized that there were many fundamental business problems better data could solve. We saw an opportunity to improve on the quality of the data behind many of today’s financial and market-based decisions.
What was even more amazing, the solution to the problem was right in front of us – U.S. Government Information. They don’t collect financial data from a sample of the population and they don’t just rely on surveys. They have complete data sets. 144 million households, 27 million for-profit public and private companies, in other words, everyone.
But, as was learned working for years with many of these same government agencies, while the information was there, it is difficult to manage, and even more difficult to integrate across agencies. That’s our secret sauce. Laboring in the labyrinth of government databases, Jose conceived of technologies and techniques that were subsequently developed and deployed at Powerlytics. These algorithms and other strategies are able to stitch together disparate datasets of structured data to create a fuller, more robust, more complete picture of households and businesses. Just the kind of information that’s been lacking.
Today, Powerlytics’s Market Intelligence Platform provides the most comprehensive, accurate and granular consumer and business financial data available. We make it easy to create precise benchmarking and market-sizing reports and to perform detailed economic, business and marketing research. This powerful information can be used to drive corporate strategy, discover potential markets, evaluate competitors, and identify risks and trends in both the business and consumer sectors.
Which brings us back to our study of the most profitable industries in the U.S. Because we have access to the most comprehensive financial data available, we were able to put to rest the myths and fallacies propagated by surveys and samples and end the debate once and for all. This attracted the attention of national publications such as Forbes, which recognized our unique capabilities. You’ll have to read my blogpost on the study to find out who “won”, and stay tuned for other studies we have planned where we intend to ensure all the facts are included to eliminate the mistakes that are bound to occur with the traditional and error prone survey approach.
Read next
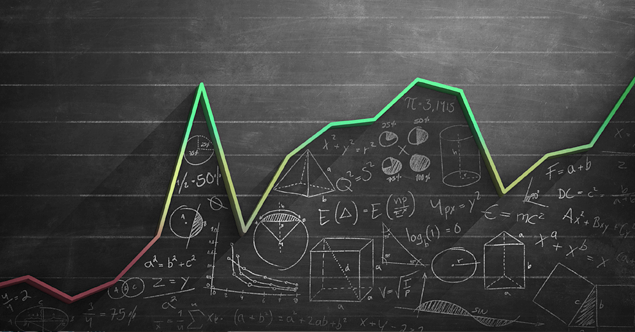
Published 21st Dec 2014
ArchivedLicensing Agreements with PolicyMap and (RE)meter Illustrate the Versatility and Veracity of Our Market Intelligence Platform
When we set out to create Powerlytics, we believed we could create a market intelligence tool with applications ranging from risk and compliance to market growth and research and analysis. I am...
Read Article