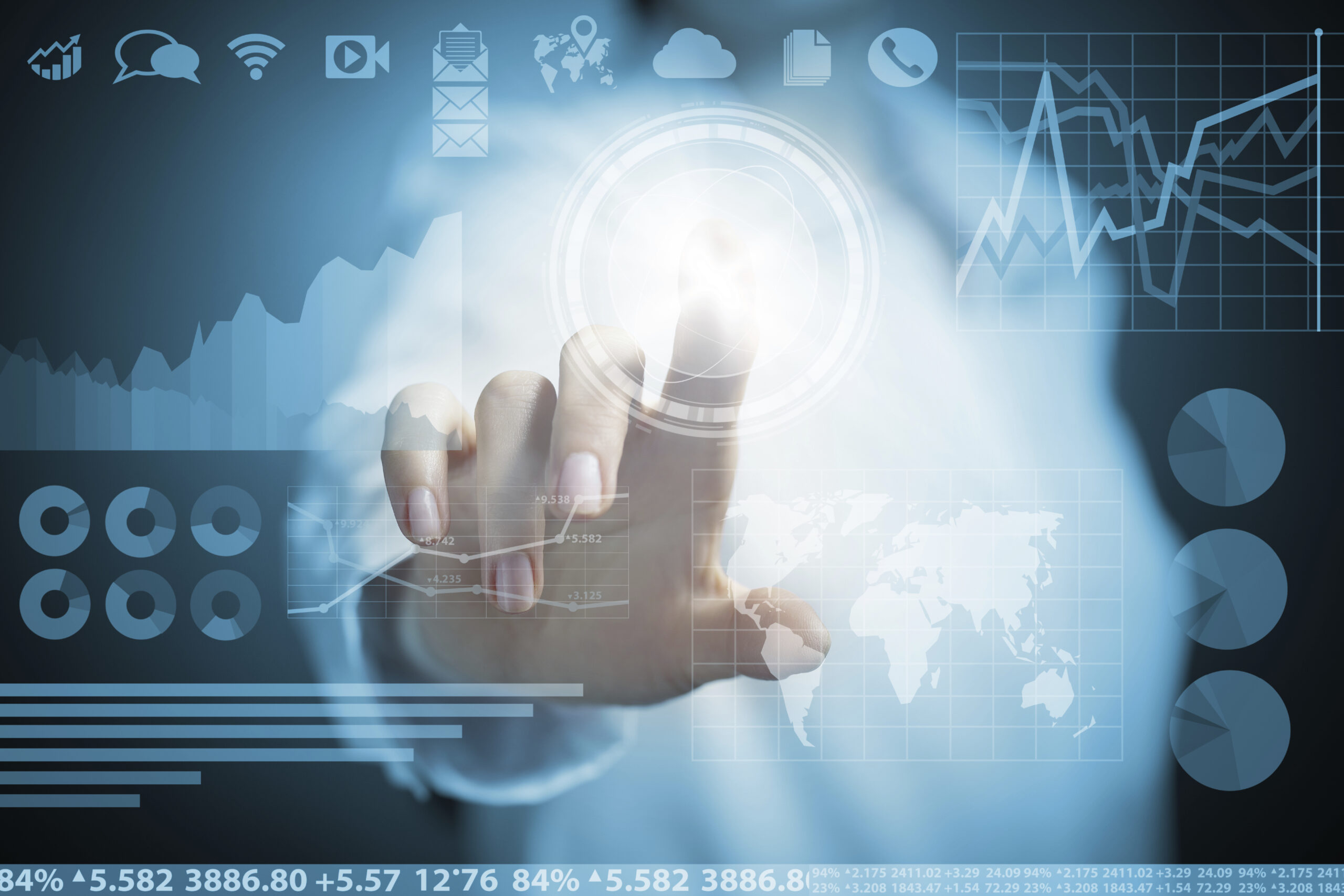
How Unreliable Data Could be Costing You Millions of Dollars a Year
Published 12th Aug 2015Garbage in, garbage out is no way to run a business. And yet that’s exactly what many insurers and financial institutions are doing. The hype of big data and the availability of information have led many to latch onto data that’s incomplete, erroneous, misleading, or just plain false. And every day you spend making decisions on partial or unreliable data is costing you.
The true cost of bad data is difficult to surmise, but some estimates say it leaches 10-25 percent of an organization’s overall revenue every year (some estimate as high as 30 percent). Every decision adds up, and if those decisions are based on the wrong information, the costs can pile up across your entire customer base.
In many cases, the problem is that financial institutions, insurers, and professional services providers place their trust in third-party or survey-based data that’s inherently flawed. Selection biases, self-reported survey information, extrapolating a sample of responses across a larger population — these flaws in much of the data used routinely for business decisions show how much data is lacking in one fundamental element: truth.
The veracity of your data is the difference between a guesstimate and an informed decision. For example, if you’re an insurer looking to market a new policy for high value homes within the Chicago area, you would be interested in looking at the demographics of wealthy zip codes that may hold potential customers. To find that information it stands to reason you might consult census data. And when you look at a wealthy zip code like 60062, the average income is $100,520 according to the 2010 census survey. But relying on a single source of self-reported information (from five years ago) is almost always misleading.
With Powerlytics’ more accurate and timely sources that go beyond self-reported information, you’d find the average income in the same zip code is actually $139,722– a 40 percent variance! That means an entirely different approach to that market including a different risk profile for the customers.
Not only can flawed data guide you down the wrong path, it also inhibits you from capitalizing on existing opportunities. While much emphasis is placed on employing data to find new customers, retaining revenue from existing customers is low-hanging fruit. But to reap what you sow, accurate data must be present to predict the behavior of your existing customers.
An insurance case study better illustrates this point. Working with a top 25 insurance carrier, we tested a selection from the more than 200 Powerlytics variables to determine if any were highly predictive of retention. The model we built inclusive of these variables was estimated to result in 0.19 percent incremental policy growth above the carrier’s original model.
While it might look small as a percentage, that incremental benefit is significant. Based on the carrier’s 2 million home-owners policies, the Powerlytics retention model could save an additional 3,800 policies. Multiplied by an average premium of $1,000, that equates to $3.8 million dollars a year.
The cost of bad data is real, and it’s adding up every day you base decisions on erroneous or incomplete information. But the flip side of risk is opportunity. You know your customers, and you have internal data. Imagine layering that with external data to deepen insight into risk and markets you serve. What more could you see? What more could you do? Driving business growth will always be a daunting task, but data veracity can be your guiding light.
Read next
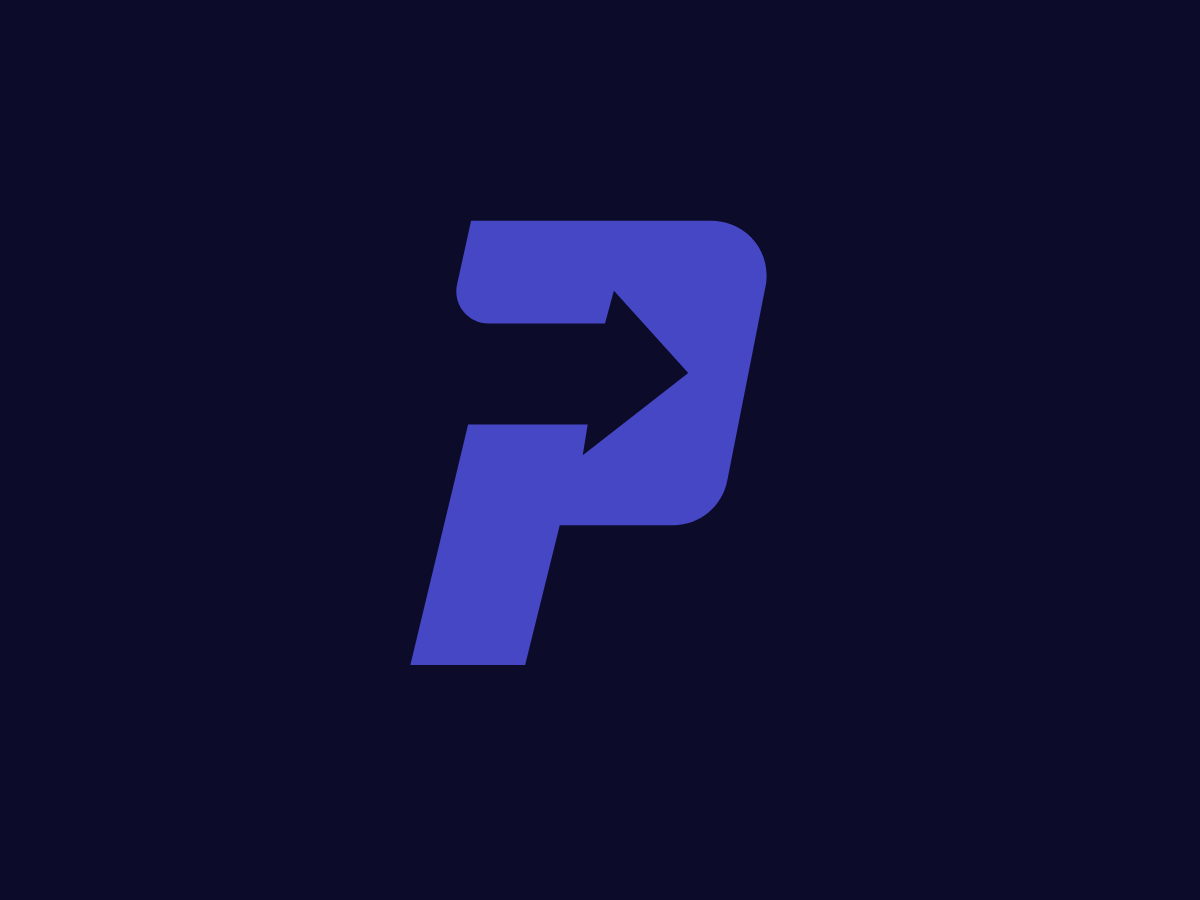
Published 16th Jul 2015
Archived7 Common Biases That Skew Big Data Results
Beware of these seven types of bias that commonly challenge organizations’ ability to make smart decisions.
Read Article